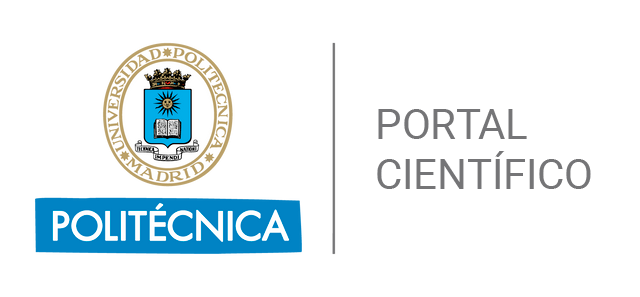
Indexed in
License and use
Optimising Convolutional Neural Networks using a Hybrid Statistically-driven Coral Reef Optimisation algorithm
Publicated to:Applied Soft Computing. 90 (106144): - 2020-05-01 90(106144), DOI: 10.1016/j.asoc.2020.106144
Authors: Martín A., Vargas V.M., Gutiérrez P.A., Camacho D., Hervás-Martínez C.
Affiliations
Abstract
© 2020 Elsevier B.V. Convolutional Neural Networks stands at the front of many solutions which deal with computer vision related tasks. The use and the applications of these models are growing unceasingly, as well as the complexity required to deal with bigger and highly complex problems. However, hitting the most suitable model for solving a specific task is not trivial. A very manually intensive and time consuming trial-and-error experimentation is needed in order to find an architecture, hyperparameters and parameters which reach a certain level of performance. Moreover, this process leads to oversized models, diminishing their generalisation capacity. In this paper, we leverage a metaheuristic and a hybridisation process to optimise the reasoning block of CNN models, composed by fully connected and dropout layers, conducting a full reconstruction that leads to lighter models with better performance. Our approach is architecture-independent and operates at the topology, hyperparameters and parameters (connection weights) levels. For that purpose, we have implemented the Hybrid Statistically-driven Coral Reef Optimisation (HSCRO) algorithm as an extension of SCRO, a metaheuristic which does not require to adjust any parameter since they are automatically and dynamically chosen based on the statistical characteristics of the evolution. In addition, a hybridisation process employs the backpropagation algorithm to make a final fine-grained weights adjustment. In the experiments, the VGG-16 model is successfully optimised in two different scenarios (the CIFAR-10 and the CINIC-10 datasets), resulting in a lighter architecture, with an 88% reduction of the connection weights, but without losing its generalisation performance.
Keywords
Quality index
Bibliometric impact. Analysis of the contribution and dissemination channel
The work has been published in the journal Applied Soft Computing due to its progression and the good impact it has achieved in recent years, according to the agency WoS (JCR), it has become a reference in its field. In the year of publication of the work, 2020, it was in position 11/111, thus managing to position itself as a Q1 (Primer Cuartil), in the category Computer Science, Interdisciplinary Applications. Notably, the journal is positioned above the 90th percentile.
From a relative perspective, and based on the normalized impact indicator calculated from World Citations from Scopus Elsevier, it yields a value for the Field-Weighted Citation Impact from the Scopus agency: 1.13, which indicates that, compared to works in the same discipline and in the same year of publication, it ranks as a work cited above average. (source consulted: ESI Nov 14, 2024)
This information is reinforced by other indicators of the same type, which, although dynamic over time and dependent on the set of average global citations at the time of their calculation, consistently position the work at some point among the top 50% most cited in its field:
- Field Citation Ratio (FCR) from Dimensions: 9.18 (source consulted: Dimensions May 2025)
Specifically, and according to different indexing agencies, this work has accumulated citations as of 2025-05-25, the following number of citations:
- WoS: 13
- Scopus: 29
- Google Scholar: 34
- OpenCitations: 27
Impact and social visibility
Leadership analysis of institutional authors
There is a significant leadership presence as some of the institution’s authors appear as the first or last signer, detailed as follows: First Author (MARTIN GARCIA, ALEJANDRO) .
the author responsible for correspondence tasks has been CAMACHO FERNANDEZ, DAVID.