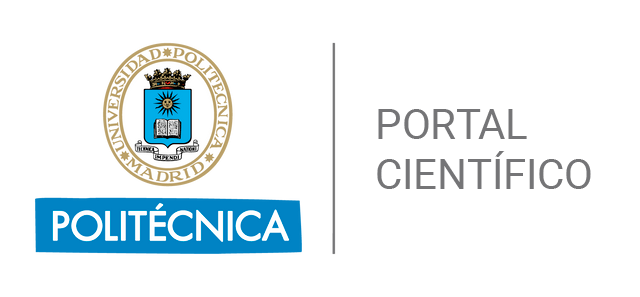
Indexado en
Licencia y uso

Grant support
This work was supported by the Major Projects of National Natural Science Foundation of China (U20A20105), National Key Research and Development Project of China (2021YFA1000103, 2021YFA1000102), National Natural Science Foundation of China (Grant Nos. 61972416, 62272479, 62202498), Taishan Scholarship (tsqn201812029), Shandong Provincial Natural Science Foundation (ZR2021QF023), Fundamental Research Funds for the Central Universities (21CX06018A), Spanish project PID2019-106960GB-I00, Juan de la Cierva IJC2018-038539-I.
Análisis de autorías institucional
Song, TaoAutor o CoautorConvformer: A Model for Reconstructing Ocean Subsurface Temperature and Salinity Fields Based on Multi-Source Remote Sensing Observations
Publicado en:Remote Sensing. 16 (13): 2422- - 2024-07-01 16(13), DOI: 10.3390/rs16132422
Autores: Song, Tao; Xu, Guangxu; Yang, Kunlin; Li, Xin; Peng, Shiqiu
Afiliaciones
Resumen
Observational data on ocean subsurface temperature and salinity are patently insufficient because in situ observations are complex and costly, while satellite remote-sensed measurements are abundant but mainly focus on sea surface data. To make up for the ocean interior data shortage and entirely use the abundant satellite data, we developed a data-driven deep learning model named Convformer to reconstruct ocean subsurface temperature and salinity fields from satellite-observed sea surface data. Convformer is designed by deeply optimizing Vision Transformer and ConvLSTM, consisting of alternating residual connections between multiple temporal and spatial attention blocks. The input variables consist of sea surface temperature (SST), sea surface salinity (SSS), sea surface height (SSH), and sea surface wind (SSW). Our results demonstrate that Convformer exhibits superior performance in estimating the temperature-salinity structure of the tropical Pacific Ocean. The all-depth average root mean square error (RMSE) of the reconstructed subsurface temperature (ST)/subsurface salinity (SS) is 0.353 degrees C/0.0695 PSU, with correlation coefficients (R-2) of 0.98663/0.99971. In the critical thermocline, although the root mean square errors of ST and SS reach 0.85 degrees C and 0.121 PSU, respectively, they remain smaller compared to other models. Furthermore, we assessed Convformer's performance from various perspectives. Notably, we also delved into the potential of Convformer to extract physical and dynamic information from a model mechanism perspective. Our study offers a practical approach to reconstructing the subsurface temperature and salinity fields from satellite-observed sea surface data.
Palabras clave
Indicios de calidad
Impacto bibliométrico. Análisis de la aportación y canal de difusión
El trabajo ha sido publicado en la revista Remote Sensing debido a la progresión y el buen impacto que ha alcanzado en los últimos años, según la agencia WoS (JCR), se ha convertido en una referencia en su campo. En el año de publicación del trabajo, 2024 aún no existen indicios calculados, pero en 2023, se encontraba en la posición 110/358, consiguiendo con ello situarse como revista Q1 (Primer Cuartil), en la categoría Environmental Sciences.
2025-04-30:
- WoS: 1
- Scopus: 5
Impacto y visibilidad social
Análisis de liderazgo de los autores institucionales
Este trabajo se ha realizado con colaboración internacional, concretamente con investigadores de: China.
Existe un liderazgo significativo ya que algunos de los autores pertenecientes a la institución aparecen como primer o último firmante, se puede apreciar en el detalle: Primer Autor (SONG, TAO) .