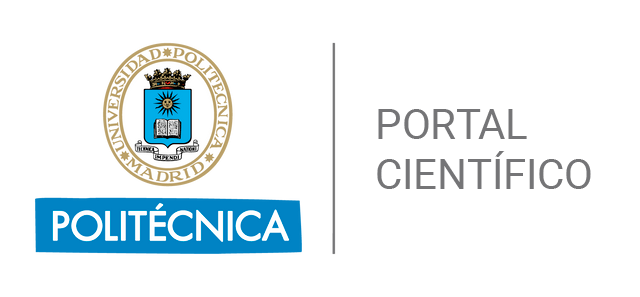
Indexado en
Licencia y uso

Análisis de autorías institucional
Riquelme-Dominguez, JmAutor (correspondencia)A machine learning-based methodology for short-term kinetic energy forecasting with real-time application: Nordic Power System case
Publicado en:International Journal Of Electrical Power & Energy Systems. 156 109730- - 2024-02-01 156(), DOI: 10.1016/j.ijepes.2023.109730
Autores: Riquelme-Dominguez, JM; Carranza-García, M; Lara-Benítez, P; González-Longatt, FM
Afiliaciones
Resumen
The progressive substitution of conventional synchronous generation for renewable-based generation imposes a series of challenges in many aspects of modern power systems, among which are the issues related to the low rotational inertia systems. Rotational inertia and the kinetic energy stored in the rotating masses in the power system play a fundamental role in the operation of power systems as it represents in some sort the ability of the system to withstand imbalances between generation and demand. Therefore, transmission system operators (TSOs) need tools to forecast the inertia or the kinetic energy available in the systems in the very short term (from minutes to hours) in order to take appropriate actions if the values fall below the one that ensures secure operation. This paper proposes a methodology based on machine learning (ML) techniques for short-term kinetic energy forecasting available in power systems; it focuses on the length of the moving window, which allows for obtaining a balance between the historical information needed and the horizon of forecasting. The proposed methodology aims to be as flexible as possible to apply to any power system, regardless of the data available and the software used. To illustrate the proposed methodology, time series of the kinetic energy recorded in the Nordic Power System (NPS) has been used as a case study. The results show that Linear Regression (LR) is the most suitable method for a time horizon of one hour due to its high accuracy to-simplicity ratio, while Long Short-Term Memory (LSTM) is the most accurate for a forecasting horizon of four hours. Experimental assessment has been carried out using Typhoon HIL-404 simulator, verifying that both algorithms are suitable for real-time simulation.
Palabras clave
Indicios de calidad
Impacto bibliométrico. Análisis de la aportación y canal de difusión
El trabajo ha sido publicado en la revista International Journal Of Electrical Power & Energy Systems debido a la progresión y el buen impacto que ha alcanzado en los últimos años, según la agencia WoS (JCR), se ha convertido en una referencia en su campo. En el año de publicación del trabajo, 2024 aún no existen indicios calculados, pero en 2023, se encontraba en la posición 65/353, consiguiendo con ello situarse como revista Q1 (Primer Cuartil), en la categoría Engineering, Electrical & Electronic.
2025-04-30:
- WoS: 4
- Scopus: 5
- OpenCitations: 2
Impacto y visibilidad social
Análisis de liderazgo de los autores institucionales
Este trabajo se ha realizado con colaboración internacional, concretamente con investigadores de: United Kingdom.
Existe un liderazgo significativo ya que algunos de los autores pertenecientes a la institución aparecen como primer o último firmante, se puede apreciar en el detalle: Primer Autor (RIQUELME DOMINGUEZ, JOSE MIGUEL) .
el autor responsable de establecer las labores de correspondencia ha sido RIQUELME DOMINGUEZ, JOSE MIGUEL.