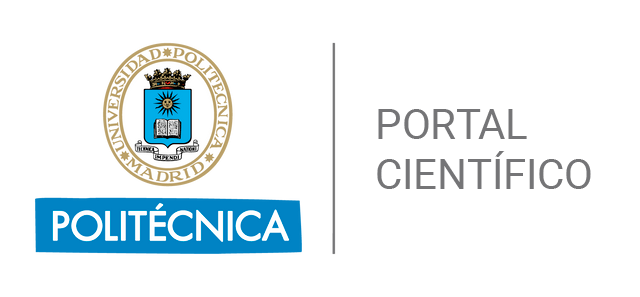
Indexado en
Licencia y uso
Citaciones
Análisis de autorías institucional
Gil-Martín M.Autor o CoautorMartín-Fernández I.Autor o CoautorEsteban-Romero S.Autor o CoautorHand Gesture Recognition Using MediaPipe Landmarks and Deep Learning Networks
Publicado en:International Conference On Agents And Artificial Intelligence. 3 24-30 - 2025-01-01 3(), DOI: 10.5220/0013053500003890
Autores: Gil-Martín M; Marini MR; Martín-Fernández I; Esteban-Romero S; Cinque L
Afiliaciones
Resumen
Advanced Human Computer Interaction techniques are commonly used in multiple application areas, from entertainment to rehabilitation. In this context, this paper proposes a framework to recognize hand gestures using a limited number of landmarks from the video images. This hand gesture recognition system comprises an image processing module that extracts and processes the coordinates of 21 hand points called landmarks, and a deep neural network module that models and classifies the hand gestures. These landmarks are extracted automatically through MediaPipe software. The experiments were carried out over the IPN Hand dataset in an independent-user scenario using a Subject-Wise Cross Validation. They cover the use of different landmark-based formats, normalizations, lengths of the gesture representations, and number of landmarks used as inputs. The system obtains significantly better accuracy when using the raw coordinates of the 21 landmarks through 125 timesteps and a light Recurrent Neural Network architecture (80.56 ± 1.19 %) or the hand anthropometric measures (82.20 ± 1.15 %) compared to using the speed of the hand landmarks through the gesture (72.93 ± 1.34 %). The proposed framework studied the effect of different landmark-based normalizations over the raw coordinates, obtaining an accuracy of 83.67 ± 1.12 % when using as reference the wrist landmark from each frame, and an accuracy of 84.66 ± 1.09 % when using as reference the wrist landmark from the first video frame of the current gesture. In addition, the proposed solution provided high recognition performance even when only using the coordinates from 6 (82.15 ± 1.16 %) or 4 (81.46 ± 1.17 %) specific hand landmarks using as reference the wrist landmark from the first video frame of the current gesture.
Palabras clave
Indicios de calidad
Impacto y visibilidad social
Análisis de liderazgo de los autores institucionales
Este trabajo se ha realizado con colaboración internacional, concretamente con investigadores de: Italy.
Existe un liderazgo significativo ya que algunos de los autores pertenecientes a la institución aparecen como primer o último firmante, se puede apreciar en el detalle: Primer Autor (GIL MARTIN, MANUEL) .